RECENT ARTICLES
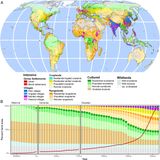
People have shaped most of terrestrial nature for at least 12,000 years
Edited by B. L. Turner, Arizona State University, Tempe, AZ, and approved March 4, 2021 (received for review November 13, 2020)The current biodiversity crisis is often depicted as a struggle to preserve untouched habitats. Here, we combine global maps of human populations and land use over the past 12,000 y with current biodiversity data to show that nearly three quarters of terrestrial nature has long been shaped by diverse histories of human habitation and use by Indigenous and traditional peoples. With rare exceptions, current biodiversity losses are caused not by human conversion or...…Edited by B. L. Turner, Arizona State University, Tempe, AZ, and approved March 4, 2021 (received for review November 13, 2020)The current biodiversity crisis is often depicted as a struggle to preserve untouched habitats. Here, we combine global maps of human populations and land use over the past 12,000 y with current biodiversity data to show that nearly three quarters of terrestrial nature has long been shaped by diverse histories of human habitation and use by Indigenous and traditional peoples. With rare exceptions, current biodiversity losses are caused not by human conversion or...WW…
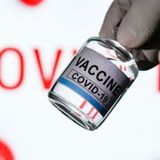
Reserving coronavirus disease 2019 vaccines for global access: cross sectional analysis
Reserving coronavirus...Reserving coronavirus disease 2019 vaccines for global access: cross sectional analysis;, 1Department of International Health, Johns Hopkins Bloomberg School of Public Health, Baltimore, MD, USA2Innovation+Design Enabling Access (IDEA) Initiative, Johns Hopkins Bloomberg School of Public Health, Baltimore, MD, USACorrespondence to: A D So (or on Twitter)Objective To analyze the premarket purchase commitments for coronavirus disease 2019 (covid-19) vaccines from leading manufacturers to recipient countries.Design Cross sectional analysis.Data sources World Health...…Reserving coronavirus...Reserving coronavirus disease 2019 vaccines for global access: cross sectional analysis;, 1Department of International Health, Johns Hopkins Bloomberg School of Public Health, Baltimore, MD, USA2Innovation+Design Enabling Access (IDEA) Initiative, Johns Hopkins Bloomberg School of Public Health, Baltimore, MD, USACorrespondence to: A D So (or on Twitter)Objective To analyze the premarket purchase commitments for coronavirus disease 2019 (covid-19) vaccines from leading manufacturers to recipient countries.Design Cross sectional analysis.Data sources World Health...WW…
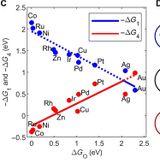
Explainable and trustworthy artificial intelligence for correctable modeling in chemical sciences
Data science has primarily focused on big data, but for many physics, chemistry, and engineering applications, data are often small, correlated and, thus, low dimensional, and sourced from both computations and experiments with various levels of noise. Typical statistics and machine learning methods do not work for these cases. Expert knowledge is essential, but a systematic framework for incorporating it into physics-based models under uncertainty is lacking. Here, we develop a mathematical and computational framework for probabilistic artificial intelligence (AI)–based predictive modeling...…Data science has primarily focused on big data, but for many physics, chemistry, and engineering applications, data are often small, correlated and, thus, low dimensional, and sourced from both computations and experiments with various levels of noise. Typical statistics and machine learning methods do not work for these cases. Expert knowledge is essential, but a systematic framework for incorporating it into physics-based models under uncertainty is lacking. Here, we develop a mathematical and computational framework for probabilistic artificial intelligence (AI)–based predictive modeling...WW…
- Total 3 items
- 1